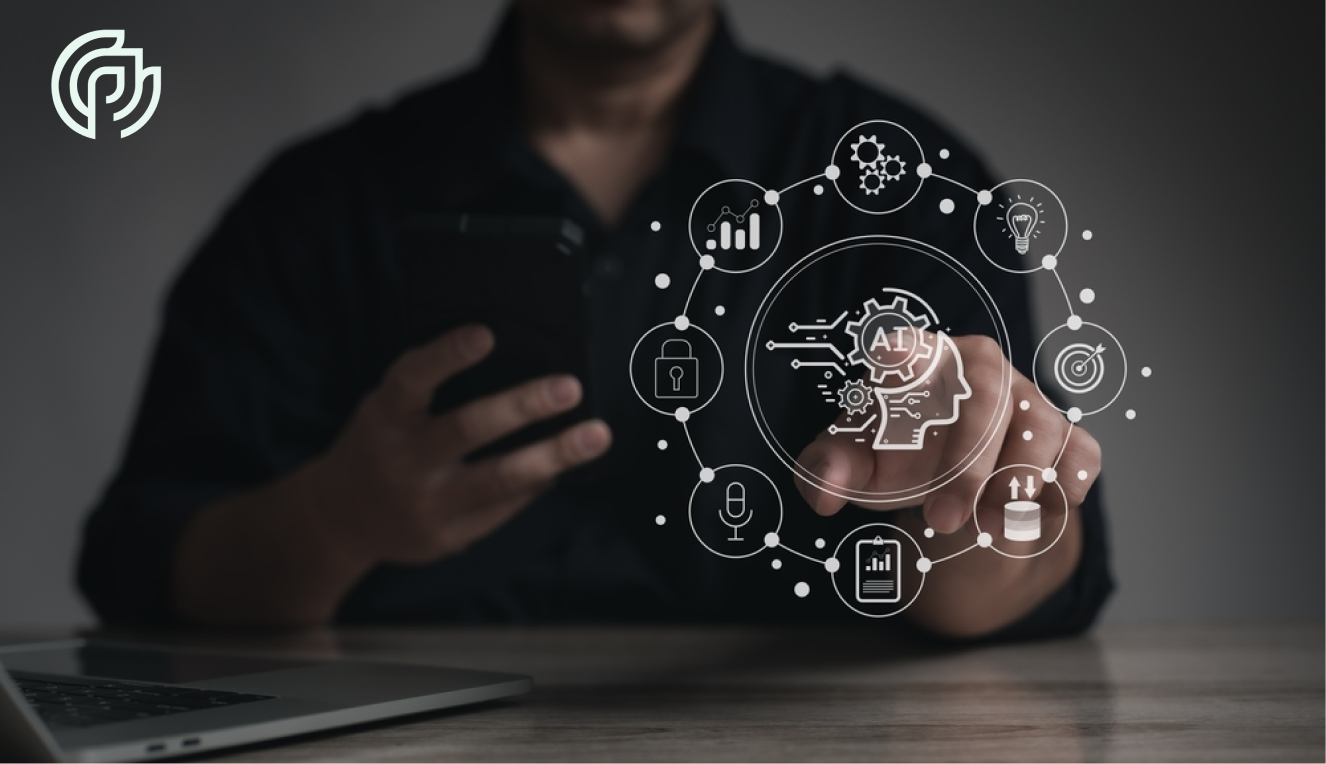
Artificial Intelligence (AI) encompasses a wide range of technologies that enable machines to perform tasks that typically require human intelligence. In the context of demand forecasting for supply chain management, several AI technologies play crucial roles in improving accuracy, efficiency, and decision-making. Here, these technologies are explained.
- Machine Learning (ML) for Demand Forecasting
Machine learning algorithms analyze large amounts of historical sales data, market trends, customer behavior, and other factors to identify patterns and predict future demand. These self-learning algorithms adapt to changing market conditions and continuously improve forecasting accuracy over time.
- Martinus, a major East European omnichannel e-commerce bookstore, partnered with FutureMargin, an AI-powered demand planning software. The results included an 84% increase in the ratio of products expedited on the day of order placement and a 14% improvement in average order fulfillment time [1].
- Amazon, one of the world’s largest retailers, uses AI to better control its supply chain. Machine learning algorithms help Amazon forecast demand more accurately, optimize inventory levels, and improve operational efficiency [2].
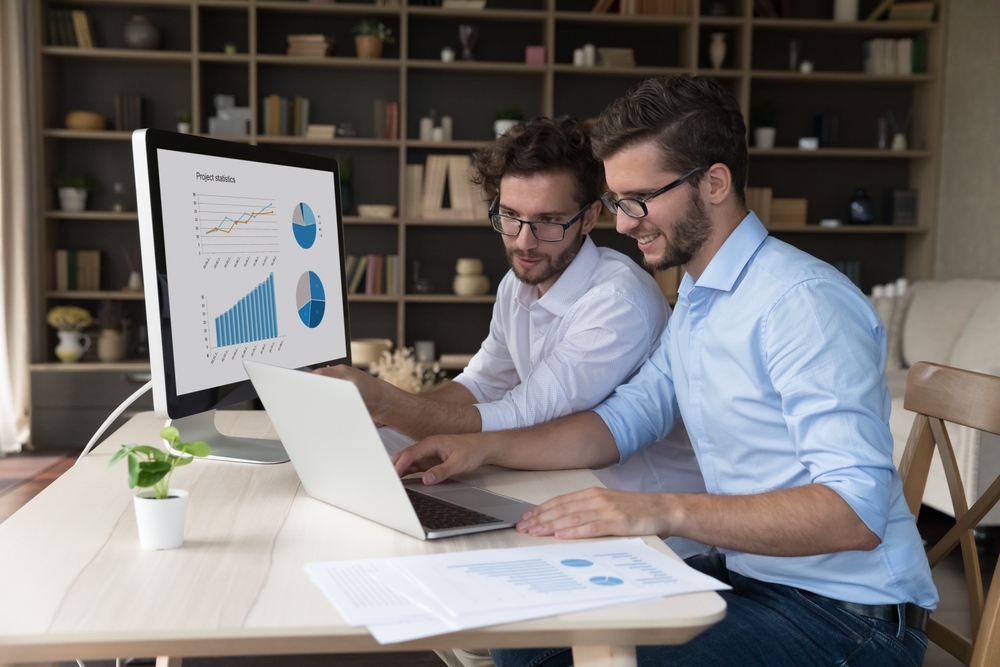
2. Predictive Analytics for Demand Forecasting
Predictive analytics techniques, such as regression analysis, time-series analysis, and neural networks, are used to forecast demand based on historical trends and patterns. By identifying factors that impact demand, companies can make accurate predictions and optimize their supply chains.
- A global consumer goods company used predictive analytics to improve demand forecasting accuracy by 20-30%, resulting in a 10-15% reduction in inventory costs and a 1-2% increase in sales [3].
- A leading automotive manufacturer implemented predictive analytics to forecast demand for spare parts. The solution improved forecast accuracy by 20%, reduced inventory costs by 10%, and increased customer satisfaction [3].
3. Natural Language Processing (NLP) for Demand Sensing
NLP analyzes unstructured data from social media, customer feedback, and other sources to identify trends and patterns that impact demand. This information is used to adjust demand forecasts and optimize supply chain operations.
- A global fashion retailer used NLP to analyze customer sentiment on social media and adjust demand forecasts for upcoming seasons. The solution improved forecast accuracy by 15% and reduced markdowns by 10% [3].
- A leading consumer electronics company used NLP to monitor customer reviews and feedback, identifying potential quality issues and adjusting demand forecasts accordingly. The solution helped reduce warranty claims by 20% and improve customer satisfaction [3].
4. Image Recognition for Demand Forecasting
Image recognition technologies analyze product images and videos to understand popularity and identify trends. By scanning online product images, social media images, and in-store images, these technologies can identify patterns related to product demand and help improve forecasting accuracy.
- A global cosmetics company used image recognition to analyze social media images and identify trending products. The insights were used to adjust demand forecasts and optimize inventory levels, resulting in a 10% increase in sales and a 15% reduction in inventory costs [3].
- A leading furniture retailer used image recognition to analyze in-store images and identify popular product combinations. The insights were used to optimize product placement and adjust demand forecasts, resulting in a 5% increase in sales and a 10% improvement in inventory turnover [3].
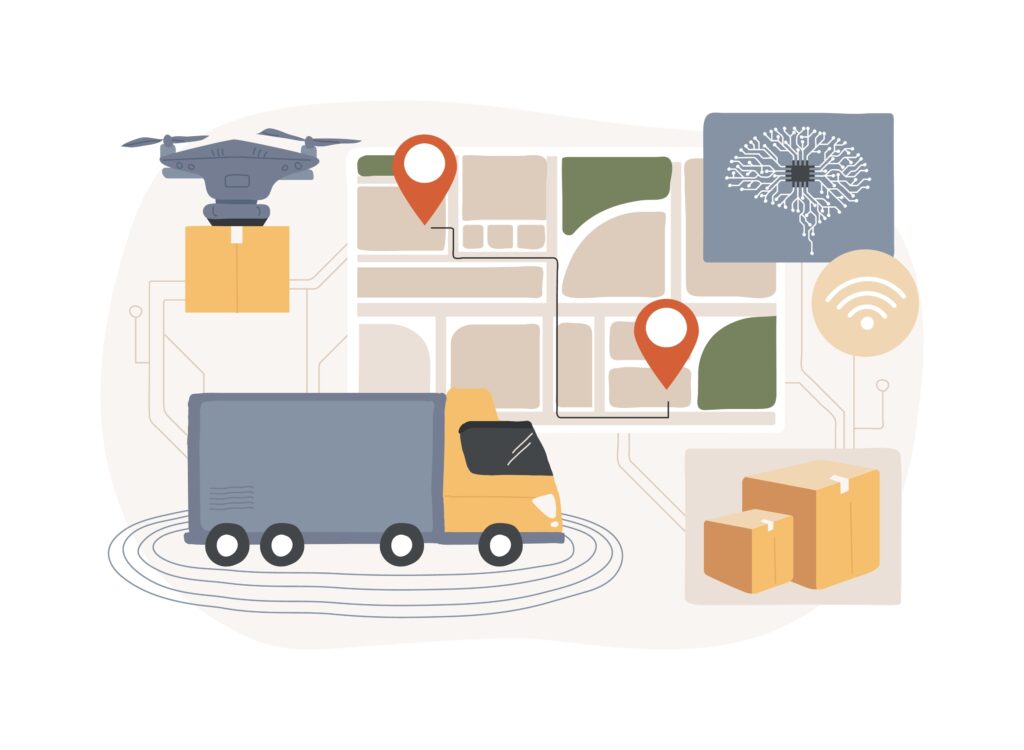
5. Internet of Things (IoT) for Real-Time Demand Sensing
IoT sensors collect real-time data on inventory levels, environmental conditions, product usage, customer behavior, and preferences. This data is analyzed using AI techniques to make accurate demand forecasts and enable dynamic pricing strategies.
- A global food and beverage company used IoT sensors to monitor inventory levels and product freshness in real-time. The data was used to optimize inventory management and adjust demand forecasts, resulting in a 20% reduction in waste and a 10% increase in sales [3].
- A leading industrial equipment manufacturer used IoT sensors to monitor product usage and performance in real-time. The data was used to predict maintenance needs, optimize spare parts inventory, and adjust demand forecasts, resulting in a 15% reduction in downtime and a 10% improvement in customer satisfaction [3].
References:
[1] “Demand Forecasting in the Age of AI & Machine Learning [2024],” AIMultiple: High Tech Use Cases & Tools to Grow Your Business. Accessed: May 01, 2024. [Online]. Available: https://research.aimultiple.com/demand-forecasting/
[2] “How will AI change demand forecasting in the supply chain?,” Transition Technologies PSC. Accessed: May 01, 2024. [Online]. Available: https://ttpsc.com/en/blog/how-will-ai-change-demand-forecasting-in-the-supply-chain/
[3] S. Tycast, “AI for Supply Chain Optimization: Improve Demand Forecasting,” AIM Consulting. Accessed: May 01, 2024. [Online]. Available: https://aimconsulting.com/insights/ai-supply-chain-optimization-demand-forecasting/